EEOB Publication - Berger-Wolf
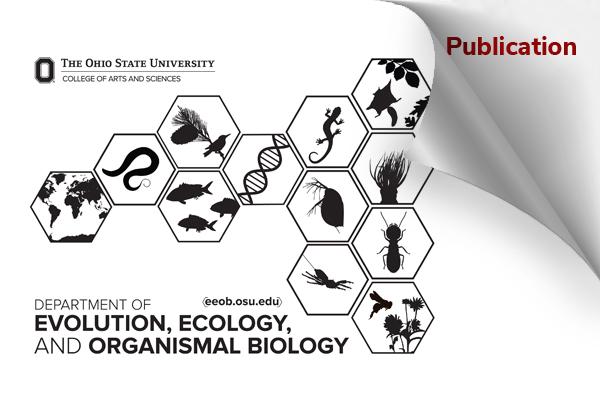
Integrating Biological Data into Autonomous Remote Sensing Systems for In Situ Imageomics: A Case Study for Kenyan Animal Behavior Sensing with Unmanned Aerial Vehicles (UAVs)
Jenna M. Kline, Maksim Kholiavchenko, Otto Brookes, Tanya Berger-Wolf, Charles V. Stewart, Christopher Stewart. 2024. Association for the Advancement of Artificial Intelligence. Link to article.
Abstract
In situ imageomics leverages machine learning techniques to infer biological traits from images collected in the field, or in situ, to study individuals, organisms, groups of wildlife, and whole ecosystems.Such datasets provide real-time social and environmental context to inferred biological traits, which can enable new, data-driven conservation and ecosystem management. The development of machine learning techniques to extract biological traits from images are impeded by the volume and quality data required to train these models. Autonomous, unmanned aerial vehicles(UAVs, are well suited to collect in situ imageomics data as they can traverse remote terrain quickly to collect large volumes of data with greater consistency and reliability compared to manually piloted UAV missions.However, littleguidanceexistsonoptimizingautonomousUAVmissionsforthepurposesofremotesensing for conservation and biodiversity monitoring. The UAV video data set curated by KABR: In-Situ Dataset for Kenyan Animal Behavior Recognition from Drone Videos (Kholiavchenkoetal. 2024)required three weeks to collect, a time-consuming and expensive endeavor. Our analysis of KABR revealed that a third of the videos gathered were unusable for the purposes of inferring wildlife behavior. We analyzed the flight telemetry data from portions of UAV videos that were usable for inferring wildlife behavior, and demonstrate how these insights can be integrated into an autonomous remote sensing system to track wildlife in real time. Our autonomous remote sensing system optimizes the UAV’s actions to increase the yield of usable data, and matches the flightpath of an expert pilot with an 87% accuracy rate, representing an 18.2% improvement in accuracy over previously proposed methods.